By Intissar Keghouche, DELFI lead, StormGeo
“All models are wrong, but some are useful,” the statistician George Box wrote in 1976. This familiar aphorism sheds light on the fact that models cannot capture the complexities of the real world. Whether we use models to study financial market mechanisms, predict optimal healthcare strategies or, for any other use case, they can be useful but never truly accurate.
The same goes for numerical weather prediction. As most of us have experienced watching the weather on TV, weather forecasts are rarely perfect. Like all forecast models, weather models are only an approximation of the world that tries to predict the conditions of the atmosphere by collecting quantitative data and relying on advanced calculation methods.
Recent technological advancements, however, promise to improve the accuracy of weather forecasting. Today, advanced machine learning techniques and increased computing power can reduce weather forecast errors, ultimately helping meteorologists develop better predictions.
This is good news for the offshore wind industry, as the weather plays a critical role in the construction, maintenance, and productivity of offshore wind farms. Wave height determines the working windows of wind farm installation vessels and limits the accessibility of wind turbines for maintenance. Lightning and thunderstorms can endanger the safety of maintenance crews working on the turbines. And strong winds can reduce the operability of cranes and thereby limit the working windows during installation.
With more accurate weather forecasts at hand, offshore wind players can efficiently reduce downtime and costs while simultaneously increasing the safety of personnel and maintenance crews.
As a global partner for the offshore wind industry, StormGeo strives to improve its weather forecasts to help offshore wind developers and operators ensure safer and more efficient wind farm installations and maintenance. That is why StormGeo has developed DELFI, a weather forecasting algorithm that leverages the power of machine learning to improve offshore wind operations and maintenance worldwide.
DELFI: Machine learning for offshore weather forecasting
DELFI (deep learning forecast improvement) is StormGeo’s machine learning system, raising the quality of weather forecasts by correcting forecast errors adaptively. It leverages a broad range of advanced machine learning techniques, from linear models to deep neural networks, to automatically improve forecast quality by learning the error patterns from the forecast systems.
Every week, DELFI compares the forecasts produced by the different methods with all available observation data and chooses the method with the highest score. That method is then used in the forecasting for the next week. The process is repeated every week, always adding last week’s observations to its verification process.
DELFI differs from traditional weather forecasting in that it reduces the need for manual intervention in position-based forecasts. Where we often needed to rely on forecasters to learn, remember and act on the model’s weaknesses in the past, DELFI now learns these weaknesses and automatically reduces most of these errors. DELFI, then, provides a more accurate starting point than any of the input forecasts.
Machine learning-based forecast improvement methods, such as DELFI, are, in principle, not that different from conventional weather forecasting. In both cases, somebody or something must understand and learn the forecasting system weaknesses and leverage this knowledge to improve the accuracy. The main difference, however, is that the machine learning system more efficiently identifies errors compared to a traditional weather forecaster. DELFI, then, provides a more accurate forecast baseline that helps the forecaster work more efficiently and make better decisions.
DELFI, and machine learning in general, not only improves the accuracy of weather forecasts but also improves efficiency by automating tasks traditionally performed by human weather forecasters. The forecaster’s role will change accordingly, moving away from detailed intervention of position-based forecasts to focus more on decision support for offshore wind clients, optimizing the forecasts, identifying potentially breaching thresholds and providing advice when observations do not match the forecasts.
In other words, DELFI reduces the subjective element of weather forecasting, which in turn creates more consistent and efficient predictions that are less dependent on the inclinations of individual forecasters. For some offshore wind developers and operators, a more objective forecast may yield more confidence during decision-making processes.
DELFI increases forecast accuracy
StormGeo has successfully used DELFI to improve weather forecasts for several offshore locations – even though the machine learning system is still in its early development stages.
For example, a multinational energy company recently leveraged DELFI to improve its understanding of metocean characteristics on one of its offshore locations in the North Sea. By relying on DELFI’s machine learning capabilities, the offshore site improved its results significantly compared to traditional weather forecasting methods.
The figure below shows an improved forecast accuracy at the offshore location (figure 1). The blue curve indicates observations, showing measured wave height for nine days during Autumn 2021. The orange line represents the numerical weather prediction model. The green line represents the DELFI forecast – and catches the variability correctly.
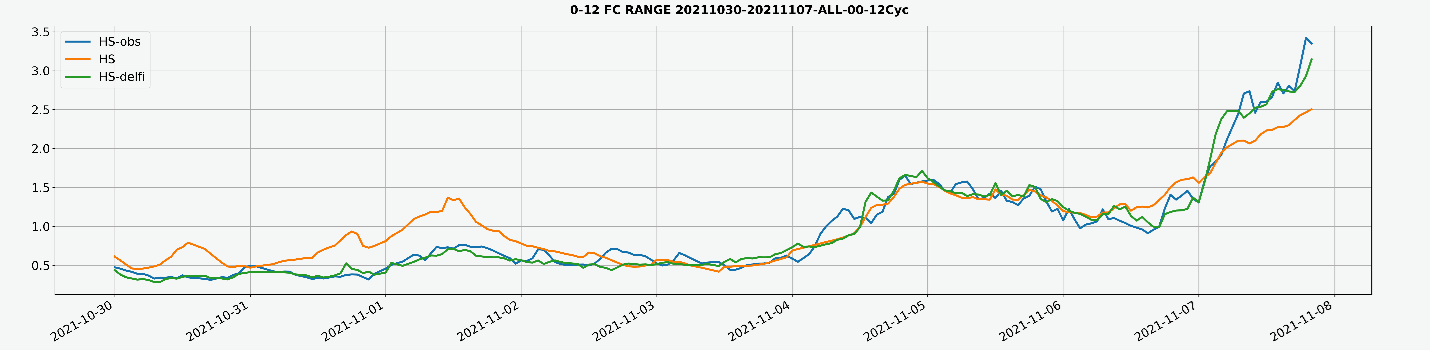
Figure 1: Time series of significant wave height forecast from 2021-10-30 to 2021-11-07. The time series of model and DELFI forecasts shown here are based on a 0 to 12 hours lead time.
For some offshore clients, the difference between 1.9 and 2.2 meters of wave height is critical to their operations and their ability to ensure safety and efficiency and reduce operational downtime. And offshore operators, in general, increasingly focus on the cost-benefit of having more accurate forecasts. Leveraging machine learning techniques helps us adapt to these changing business needs by increasing weather forecasts’ quality, accuracy, and efficiency.
A bright future for offshore weather forecasting with machine learning
The first use cases for DELFI are promising, and the system will keep on improving and extending to improve forecast quality in even more industries and for more advanced situations. And DELFI will only enhance its accuracy as it gets more use cases to learn from. StormGeo currently trains DELFI to improve the forecasts weekly, using a full range of machine learning techniques, as new and updated observation data from offshore wind farms and other offshore installations come in.
Once implemented, DELFI can result in significant weather forecast accuracy improvement, ultimately helping offshore wind developers and operators safely plan their operations and improve operational spending.
Dr. Intissar Keghouche is a senior scientist with expertise in operational oceanography, metocean forecasting, and statistics. She holds a Ph.D. in physical oceanography from the University of Bergen. Currently, she leads DELFI, a project which combines observations and numerical weather predictions to enhance the skills of weather forecasts using ML techniques.
Filed Under: Featured